Can artificial intelligence be biased?
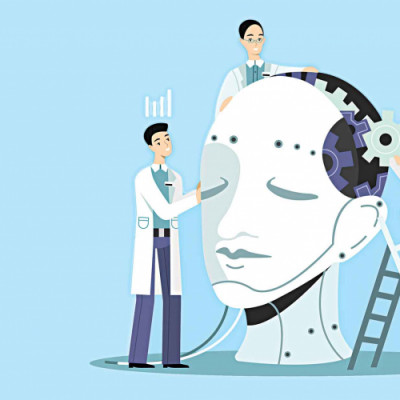
Algorithms are everywhere. It's guiding our internet search results, removing the guesswork from our online shopping experience, assisting us in selecting the next Netflix show and even in the ads we see on Facebook.
Algorithms are nothing more than math and code. However, they are created by humans and rely on our data. Since humans are susceptible to error and prejudice, the algorithms they create may have errors too. Depending on who designs them, how they are built, and how they are actually used, these systems may be biased. Actual biases are imitated or even overstated by AI systems. This is known as algorithmic bias.
Bias isn't always a bad thing. Our minds are attempting to locate trends in data in order to save time. This does not become a concern until we fail to recognise exceptions to patterns or begin to treat certain groups of people unfairly.
As a result, it is important to understand the distinction between bias, which we all have, and discrimination. Understanding algorithmic bias will benefit us in the future if it is used in negative or discriminatory ways.
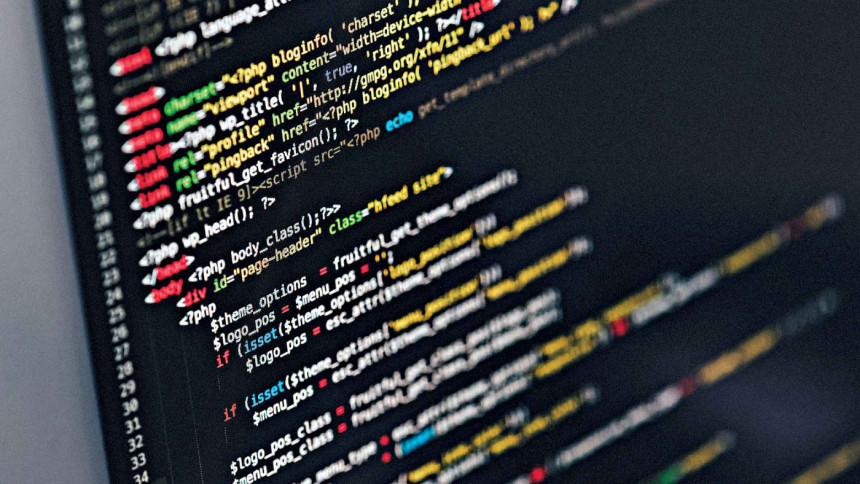
CURRENT BIASES ARE REFLECTED IN THE DATA
In 2019, Facebook began allowing advertisers to target advertisements based on gender, race, and religion. For example, women were prioritised in job advertisements for nursing and secretarial positions, while janitors and taxi drivers were mostly advertised to men, especially men from minority backgrounds.
Also, when we search the term "nurse" in Google Images, images of female nurses appear first, while the opposite happens for the word "programmer". This is because the AI was trained on news/books that correlated these occupations with a particular gender. We can see how implicit data stereotypes are ingrained in search engine AI.
What happens next is that an AI hiring system discriminates against people who do not fit the stereotype. A resume for a nursing role with the words "Male chess club captain" in it may be rejected by the AI system because it is not something associated with the average nurse. It may throw out qualified programmer resumes from people who attended women's colleges, because most programmers haven't.
A real-life example of how training data can produce sexism in an algorithm, is when Amazon trained their AI model using historical data from the previous ten years. Since there was a male domination in the tech sector and men made up 60 percent of Amazon's staff, historical data contained discrimination towards women. As a result, Amazon's hiring method wrongly learned that male applicants were preferable and downgraded female applicants. So, the machine eventually learned to discriminate against women.
PREDICTION ACCURACY
The training data may not have enough examples of each class, which can affect prediction accuracy. Many facial recognition algorithms are trained on data that contains far more faces of white people, than any other race.
A few years ago, a passport photo checker was created with an AI system which alerts the user if the person in the photo has blinked, so they can take the picture again. However, the machine continued to produce errors when presented with images of Asian people.
The algorithms will have blind spots if there is insufficient data. This is where the issue of algorithmic bias enters the picture. In certain cases, a group of people decides what data set the computer will learn from. So, if a camera company teaches its AI to identify open eyes by using Caucasians as primary learning examples, it is easy to see how the blink detection would be triggered when an Asian person steps into the frame.
DATA TAMPERING
This is when a group of people purposefully tamper with training data. For example, in early 2016,Microsoft released Tay, an AI chatbot that was expected to engage in intelligent conversations with Twitter users. Tay was trained on direct Twitter chat threads. The project would demonstrate the promise and potential of conversational interfaces powered by AI.
Tay began tweeting aggressive, sexist, and discriminatory tweets only 12 hours after it was released following a "coordinated assault by a subset of citizens." The fact that AI can be manipulated means we should treat algorithmic predictions with caution.
If algorithmic bias is not tackled, it may contribute to the amplification of human biases. Humans seem to trust the judgment of AI algorithms because they believe software isn't biased, oblivious to the fact that those decisions are already representing their own biases. As a consequence, we'll embrace AI-driven decisions and generate more flawed data for those algorithms to "improve" on.
More training data on protected groups, such as ethnicity, gender, or age, may be needed if we want less biased algorithms. Examining an algorithm's recommendation for protected groups might be a good way to search for discrimination. At the same time, these questions still arise. Can algorithms really produce total equality? And who is responsible for ensuring that the corporations and individuals who build these programs do so in the spirit of social equality?
References
1. TechTalks (2018, March 26). What is algorithmic bias? – TechTalks.
2. Forbes (2020, February 7). Biased Algorithms Learn From Biased Data: 3 Kinds Biases Found In AI Datasets.
All Nashrah cares about is smashing the patriarchy. Help her at nashrah.haque01@gmail.com
Comments