Meta's new AI model can evaluate other AI models
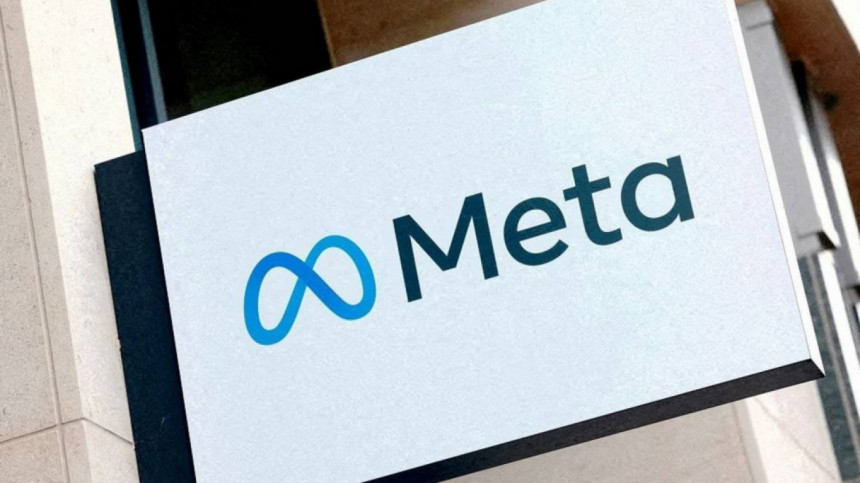
Meta has introduced a new AI model, the 'Self-Taught Evaluator (STE)', which is designed to evaluate other AI models' work with minimal human involvement.
This development aims to streamline the process of AI training by allowing AI systems to check their own outputs, potentially reducing the need for costly and time-consuming human feedback, according to an official blog post by Meta.
The STE model uses a 'chain of thought' reasoning method, where complex problems are broken down into smaller, logical steps, leading to more accurate answers in fields like science, coding, and math, explains the blog post. What distinguishes this model is its use of entirely AI-generated data during training, bypassing human input for model evaluation.
This method contrasts with traditional methods like Reinforcement Learning from Human Feedback (RLHF), which relies on human annotators to label data and verify complex answers. Meta's approach with STE removes the need for human intervention at this stage, says the tech giant.
Meta researchers suggest that the STE could pave the way for autonomous AI systems capable of learning from their own mistakes. These systems could self-evaluate, making the development of AI more efficient and scalable. Meta's tests show that the STE outperforms models trained with human-annotated data, such as GPT-4 and Llama-3.1, while operating significantly faster.
In addition to the Self-Taught Evaluator, Meta released updates to other AI tools, including improvements to its image-identification model and new datasets to assist in discovering inorganic materials. Meta is releasing these models to the public, encouraging further exploration of AI self-evaluation methods, says the company.
Comments