The pitfalls of AI: Explained
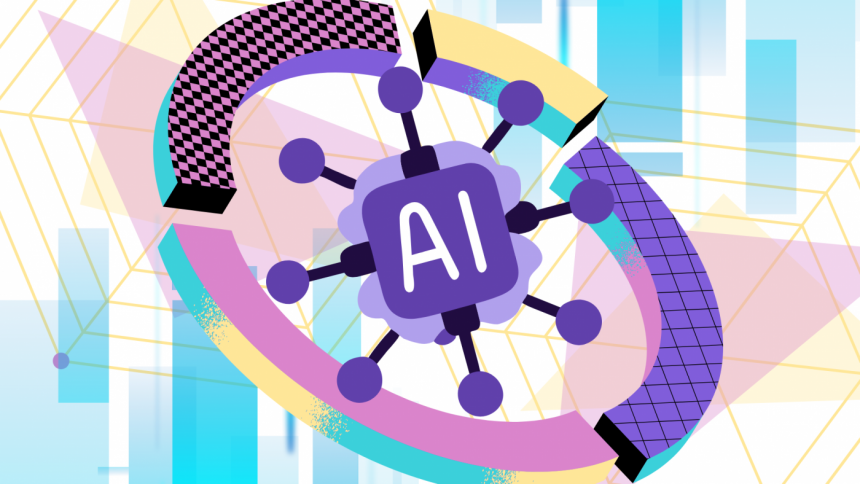
When I first came across artificial intelligence (AI), my initial thought was, "Finally, a tool that can help me with anything I need." Now, looking at how it has evolved and been adopted by humans, it's astonishing to see how AI has revolutionised industries, streamlined operations, and even attempted to emulate human creativity.
But alongside these advancements, an unexpected challenge has surfaced – AI hallucinations, a phenomenon as intriguing as it is complex.
Initially, the idea of AI hallucination reminded me of Christopher Nolan's Inception and Memento, movies where characters grappled with realities and false flashbacks. However, the concept of AI hallucination has no cinematic touch.
AI can sometimes be incorrectly decoded or lack an identifiable pattern. In other words, AI might "hallucinate" responses and provide false information due to gaps in its training data or flawed pattern recognition.
I experienced an AI hallucination when I asked an AI tool to explain a math solution that it claimed was correct. After I questioned its accuracy, however, the AI rephrased its original solution and gave me a different answer.
While it's true that AI can make mistakes, it made me wonder: if such errors can occur in something as trivial as mathematics, what about more critical areas like medical diagnosis? Research shows that AI systems that analyse medical images may incorrectly classify nodules as cancerous, resulting in unnecessary invasive procedures and emotional distress.
AI hallucination is, of course, a significant issue, but it is not the only pitfall in AI usage. There are more such pitfalls that we, as users of this technology, might encounter daily.
Inaccurate summary
AI is widely used to summarise complex topics or news pieces, and users often encounter errors here as well. Recently, AI-integrated summaries on phones or laptops have been found to present misleading summarised information, which can cause misunderstandings. For instance, the recently released iPhone 16 phones have repeatedly exhibited instances where their built-in summarisation tool provided inaccurate information, frequently omitting key details or misrepresenting the overall news.
This issue highlights a broader problem – AI-generated summaries lack the nuanced understanding that humans bring to content analysis. Users who blindly trust such summaries risk being misinformed.
Bias in decision-making
AI models that are trained on biased datasets may unintentionally reflect bias. For example, job applications powered by AI systems have sometimes unfairly filtered out resumes from candidates who have certain ethnic names or specific educational backgrounds. These biases stem from historical inequities present in training data. Without active intervention during development, AI may perpetuate systemic discrimination.
Bias in data labelling
Data labelling is a crucial step in AI development that involves human workers, which can introduce biases based on their background and interpretations. For instance, image recognition softwares trained primarily on Western datasets may struggle to accurately identify people with darker skin tones or recognise clothing styles from other regions.
Labellers are, of course, made to adhere to clear guidelines. Unfortunately, cultural and contextual differences may sometimes result in unintended consequences. That, in turn, means that biases still manage to find their way into the training data, leading to biased outcomes or results generated by the AI.
Who bears the responsibility?
As errors in AI tools remain unresolved, who bears the responsibility for this?
The challenge is significant, but there are initiatives both developers and users need to take so that they can navigate the pitfalls.
Developers play a critical role in creating transparency and accountability in AI systems. Building tools that clearly explain their decision-making processes creates public trust and helps users better understand AI outputs. Additionally, testing and independent ethical oversight are essential to identify biases and inaccuracies before deployment to ensure that the AI systems align with societal values and remain fair in their applications.
On the user end, critical thinking skills are key to responsibly using AI content. Users need to understand AI's limitations and cross-verify information with reliable sources rather than solely relying on AI-generated responses. That way, they can mitigate the impact of misinformation and AI hallucinations.
We must navigate the complexities of AI with foresight, similar to how Nolan's characters had to navigate the lines of dreams and realities. The impact of AI is still unfolding, and it's up to all of us – users, developers, and policymakers – to ensure that it's a future of progress, not peril.
References:
1. The Cureus Journal of Medical Science (2024). Revolutionizing healthcare: Qure.AI's innovations in medical diagnosis and treatment.
2. BBC (December 13, 2024). AI-integrated tools mislead users in news summaries.
3. Bloomberg (2024). AI hiring tools and racial bias: An investigation.
Nusrat Meherin Faiza is a writer, tutor, and chronic overthinker. Reach out to fuel her overthinking at nmfaiza15@gmail.com
Comments