Curbing money laundering through AI
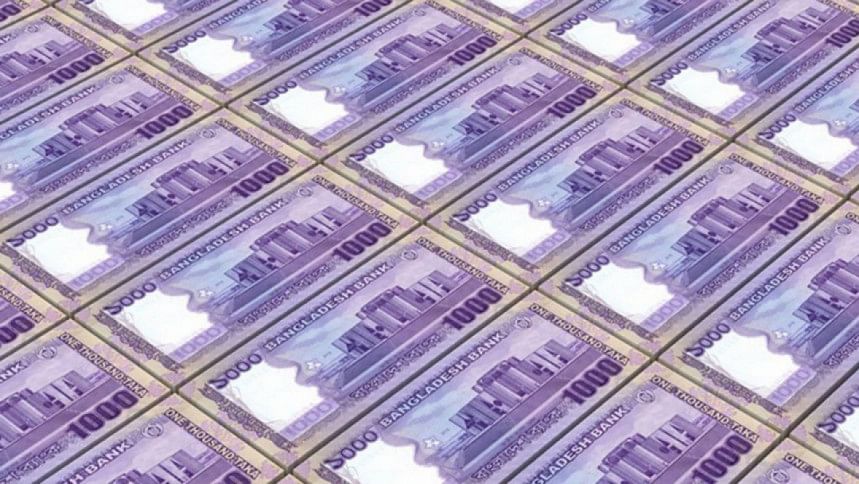
East India Company colonised Bengal in 1757 when India's contribution to the world's GDP was about 27 per cent and Bengal (Suba-E-Bengal) was one of the wealthiest places on Earth.
In 1947, when the British left, Bengal became one of the poorest places. This happened due to rampant loot and one-way transfer of wealth.
The transfer of money is still going on but the actor this time is our own people. About $7.5 billion is being transferred overseas each year, 87 per cent of which happens through trade-based money laundering (TBML).
Corruption and money laundering are inextricably linked through myriad activities involving many agencies. Prevention must follow a "Whole of Government" approach encompassing information exchange amongst different agencies.
"Follow the money" is a strategy that has been proposed by the Financial Action Task Force of the G7 countries in controlling crime and money laundering. Many law enforcement agencies of the developed world now host special units responsible for pursuing not malefactors, but bank accounts, investment portfolios, houses, and cars.
Justice systems are made responsible for managing such sequestrated assets until they can be forfeited and sold. If the wealth of people could be monitored in Bangladesh, the acquisition of black money and their subsequent trafficking could have been traced. An anti-money laundering (AML) system powered by artificial intelligence (AI) can achieve this.
The prerequisite is the digitalisation of information (data) of various organisations. Some agencies such as the Credit Information Bureau, the Election Commission and banks already have systems capable of digitally exposing data.
Other agencies such as the income tax department, the land registry departments, customs, immigration, the Anti-Corruption Commission, share-trading agents, departments with publicly funded projects, and money exchange agents can provide relevant data digitally. This is not a comprehensive list - there can be more.
The proposed AML system has (1) a database and (2) an AI component. Data would be extracted from various agencies on a daily basis and saved in a central database. The system will also collect information from people, newspapers and social media.
Data from various sources would be linked together by the national identification or tax identification number, enabling the system to correlate information from different sources and track down suspicious activities.
The decision-making process of the system must be fully automated to keep the human factor out. This is vital since millions of dollars can't be stolen unless corrupt officials are involved.
The AI component would use technologies such as machine learning, neural networks, rule-based processing and natural language processing to go through billions of data and run millions of scenarios to come up with decisions that would mimic human intelligence.
The system will run routine programmes to monitor the wealth of citizens, review foreign trades, investigate cases referred by different agencies, monitor the cost of publicly funded projects, and evaluate information gathered from the public, newspapers and social media. In that process, the system will identify TBML attempts, financial frauds, tax evasions, and corruption. Here is a hypothetical example:
During routine observation, the AML system detects a letter of credit (LC) with an inflated price. It senses a case of over-invoicing and decides to conduct an investigation in depth.
The system finds that a few years back the LC opener had a big public-funded project. Subsequently, the total asset of LC opener's wife increased 100 times. Her assets include cash in different bank accounts, company shares and real estate properties, and none of these was declared in her tax return.
Hence the AML system marks that as a case of corruption, tax evasion and attempted TBML. In this investigation, the system matched the relevant information from all departments with public-funded projects, customs, banks, share-trading agents, the land registry department, and the tax department. The whole investigation was completed in minutes.
The scope of the AML system is very large. The agile software development methodology is a process of building such systems incrementally. A minimum workable solution is built quickly, which is gradually expanded over a period of time.
The first version of the AML system can integrate information and scenarios of the Credit Information Bureau of Bangladesh and help commercial banks in identifying customers, loan defaulters and verifying clients' credit worthiness.
The Bangladesh Financial Intelligence Unit has guidelines for the prevention of TBML. These guidelines can be the starting point in crafting the initial set of rules and scenarios.
Once programmed, banks would be able to send all trade-related transactions to the system for identifying suspected TBML cases. Data from other agencies and their relevant rules and scenarios can be included subsequently until a fully functional AI-powered AML system comes into being.
Many experts claim that big corruptions in Bangladesh happen by defaulting bank loans, tax evasions and misappropriating publicly funded projects. The AML system can first look into these areas on a priority basis.
The author is an IT specialist and certified professional of the Australian Computer Society.
Comments